Gen AI seems easy. That’s what makes things so difficult
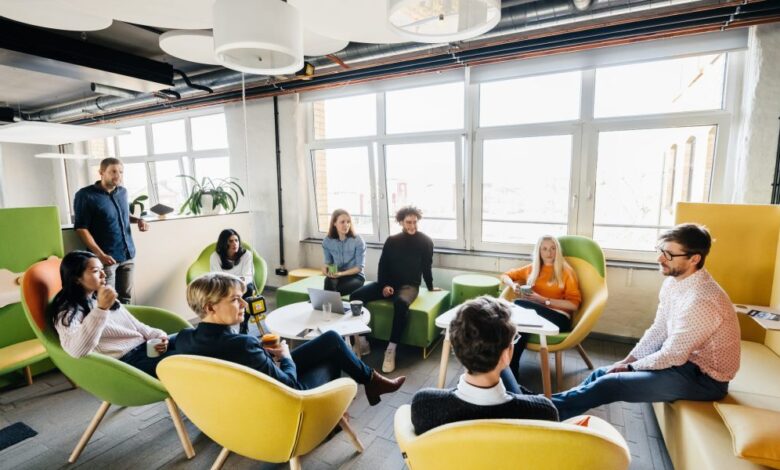
This commentary is from McKinsey & Company, a Knowledge Partner of Fortune Global Forum. Rodney Zemmel is a senior partner in McKinsey & Company’s New York office and global leader of McKinsey Digital. He is co-author of Rewired: McKinsey’s guide to excelling in the digital and AI age.
The natural language capabilities created by AI are so user-friendly that even CEOs, who are not typically early adopters, are experimenting with it. Less than a year after AI genomics-based tools exploded in late 2022, a quarter of C-Suite executives have used it already.
Widespread interest in AI generation has created a huge wave of use cases and experiments—and trouble. Such efforts are relatively easy to implement but can consume resources without creating much value.
To escape this pilot purgatory, the top priority must be connecting AI generation to business outcomes. Here are four ways CEOs can make it happen.
Focus on something important. As gene AI becomes available to more pilots, it may look like a technology in search of a problem. However, meaningful change will happen when AI generation targets an area large enough to make a difference, such as the customer journey or a functional area. For example, McKinsey worked with financial services giant ING, which created a generation of AI-powered solution Their language and data capabilities have enabled them to respond to customers with precise solutions. That improved service also frees up agents to deal with more complex issues.
Build a technology roadmap to lead the business. Gen AI has so many unknowns that there needs to be a central team, encompassing all relevant capabilities, including risk, legal, compliance, finance, HR and strategy, to develop protocols and standards. That effort must start with the CEO and C-Suite agreeing on what needs to be done. The CEO then needs to work closely with the chief information officer or chief technology officer (CIO or CTO) to translate that commitment into a concrete roadmap that guides how the company operates. Of course, transforming a domain isn’t just about next-generation AI applications; Process digitization and other forms of AI will also be involved. If applications are built around reusable modules, they can also be applied to many types of problems in the future.
Build a talent bench. Building a talent pool is non-negotiable. Partnering with external vendors, such as senior engineers who have built generative AI products, can be an important part of a generative AI strategy. But there also needs to be a greater focus on internal talent—not just across tech teams. Those on the business side also need to understand what generation AI can and cannot do.
For example, companies can upskill their data engineers to learn multimodal processing and vector database management, while data scientists can develop Quick technical and deviation detection skills. And it’s important to retain these professionals. A recent McKinsey survey of nearly 13,000 employees found that 51% of generation AI creators and heavy users plan to leave their roles within the next three to six months. Compensation is always important, but talented people are more likely to stay at a company if they can develop their skills, take on meaningful initiatives, and have opportunities for advancement.
For example, McKinsey worked with Singapore’s DBS Bank, successfully completes digital transformation, and found a winning ratio of 80% outsourced talent and 20% outsourced talent. This combination allows the organization to move faster and make decisions faster. The principle is clear: Greatness cannot be outsourced.
Focus on what’s important. Businesses are using a lot of oxygen to decide which large language model (LLM) to use. But all the new generation LLMs can do amazing things. It’s more important to put in the right efforts, such as context engineering, security, governance, and ensuring that technology upgrades support AI generation at scale. This may sound obvious, but many pilots are placed in protected environments that do not reflect reality on the ground.
Improving the data needed for specific solutions can have a huge impact on the quality of output. So does investing in an orchestration tool: Gen AI requires a lot of interaction and integration between models and applications. The application programming interface (API) gateway is a critical component of this orchestration capability because it mediates access and enforces compliance. A good API not only helps reduce risk but also gives teams confidence.
Performance gap between leaders and laggards Digital technology and AI are expanding, with leaders seeing much better financial performance. If that trend spreads to the AI generation, laggards may fall even further behind.
It’s certainly possible to capture real value from AI generation, but it’s much harder than you might imagine — partly because it seems so easy. It just doesn’t.
The views expressed in Fortune.com reviews are solely those of the author and do not necessarily reflect the views and beliefs of Luck.